The digital twin simulator model is basically a digital representation of the entire railway, with all its variances and oddities
Cultural exchange: collaborative working between GDX, GA and Mitsui
Toshiba engineers spent five months collecting data on GA’s existing timetable, the acceleration and braking performance of its rolling stock, and detailed information about the position of signals, curves and the gradient of the line, much of it courtesy of Network Rail (NR).
“The first year of the trial involved the engineers from TDX learning about how the UK rail system works, its culture, and making sure NR, as the asset owner of the track and signalling, was engaged,” says Palmer. “We had tremendous support from NR in getting access to route and signal diagrams.”
In addition to hard figures on assets and infrastructure, the TDX teams ventured out into the field in order to make themselves aware of potentially important human factors not present in the data.
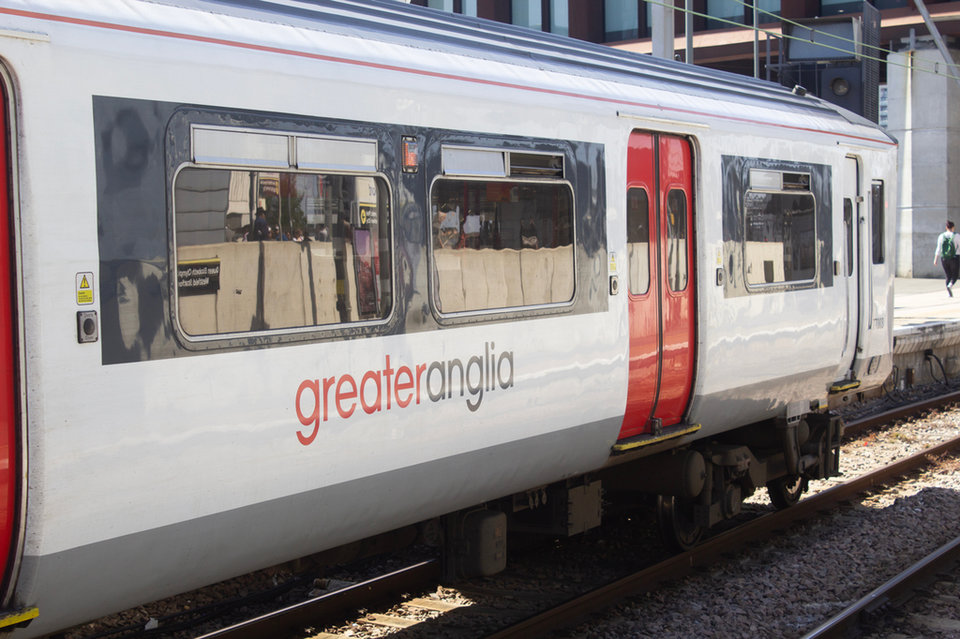
Image: Diana Vucane | Shutterstock.com
“Not everything on the railway is recordable or purely data driven,” says Palmer. “Driver behaviour – how they respond to yellow signals, for example – is very difficult to model, as is the way passengers interact in or outside major stations such as Liverpool Street. It is very difficult to pull that detail out of the data, especially when you maybe don’t appreciate all the oddities of the UK rail industry.”
GA encouraged TDX to take rides in train cabs, and talk to station staff, as well as driver managers and trainers, in order to get a feel for what actually happens on the network on a day to day basis.
Powered by innovation: inside the GDX simulator
The result is a digital twin that uses AI to organise and consolidate data that the railway industry historically kept in silos – such as track infrastructure, rolling stock performance, timetables, and rule and regulations – in order to accurately reproduce a real-world train operations environment within a cyberspace.
Operated by a TDX team in Japan, the simulator alters calling patterns, train timings and platform allocations to make the timetable more efficient and robust, improving punctuality. The adjustments are checked to make sure they are feasible and, if accepted by GA train planners in the UK, inputted into the real-world timetable. The more data entered into the system, the more accurate the output.
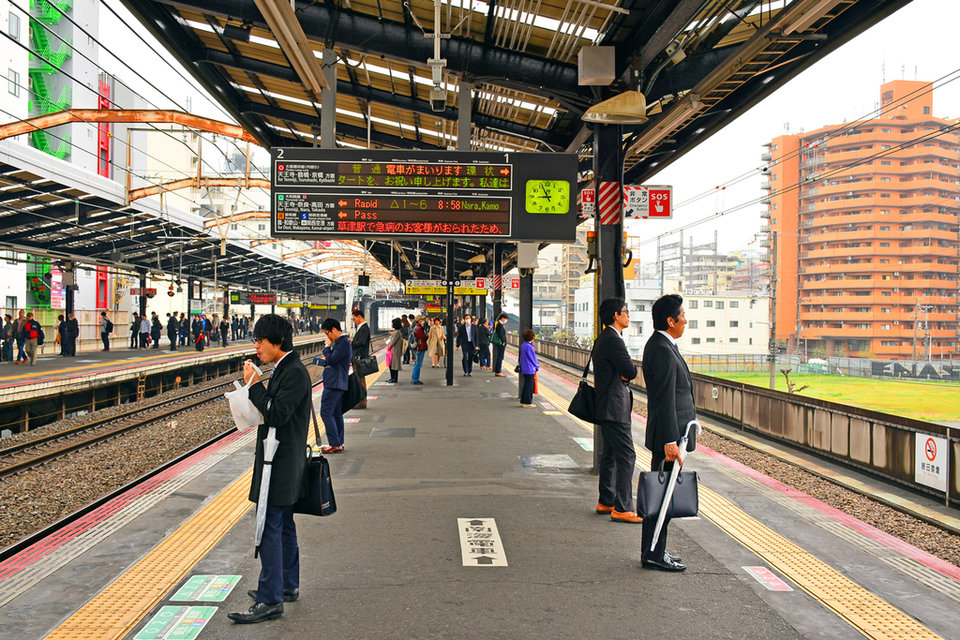
Digital twin technology has already been proven to improve punctuality in Japan. Image: imwaltersy | Shutterstock.com
“Before now, there was nothing available that allowed us to understand the performance impact of a timetable and whether it was going to cause timing issues or conflicts – the digital twin helps us to solve that problem and understand the benefits a timetable change could bring,” explains Palmer.
“By understanding a scenario before and after it occurs, and making a better decision around the performance impact, we can develop a human understanding of the railway, passenger interaction and behaviour, and dispatch arrangements. This is not about replacing experienced train planners who develop timetables; it is about giving those staff the opportunity to do more with their time.”
The simulator uses AI to organise and consolidate data that the railway industry historically kept in silos